Journal is indexed in following databases:
- SCOPUS
- Web of Science Core Collection - Journal Citation Reports
- EBSCOhost
- Directory of Open Access Journals
- TRID Database - Transportation Research Board
- Index Copernicus Journals Master List
- BazTech
- Google Scholar
2023 Journal Impact Factor - 0.7
2023 CiteScore - 1.4
ISSN 2083-6473
ISSN 2083-6481 (electronic version)
Editor-in-Chief
Associate Editor
Prof. Tomasz Neumann
Published by
TransNav, Faculty of Navigation
Gdynia Maritime University
3, John Paul II Avenue
81-345 Gdynia, POLAND
e-mail transnav@umg.edu.pl
Degradation Data Self-Analysis Layer for Integrated Maintenance Activities
1 AGH University of Science and Technology, Kraków, Poland
ABSTRACT: Reliability-oriented approach based on Monte Carlo simulations is a well-established methodology for coordinating maintenance activities of any technical system. Usually, coordination is conducted using holistic performance indicators, which are obtained from the convolution between the stochastic system availability and the system service required in a time horizon of t. Specifically, the system stochastic availability modeling is composed of the degradation process due to the system operation and the planning of the maintenance activities needed to keep the system operating at the desired standards. In the case of the degradation modeling process, given its random nature, it is addressed with predictions, which in practice, consist of generating random samples of the stochastic degradation processes from probability distributions, and the parameterization is usually estimated by fitting the distributions to historical degradation data for each technical component considered. Crucial to forecasting accurate performance indicators is the use of up-to-date information, i.e., the self-update of historical degradation data. In this paper, to address accurate performance indicators, we propose using the machine learning approach to update the adaptable model layers affected by changes in the degradation data. The paper's case study is an overhead crane system of a hot rolling mill process in a steel plant, which operates under hazardous conditions and continuously. We focus on overhead cranes because they are critical components of production processes. The paper's subject is validating the performance of a self-analysis layer, which processes the degradation data of the analyzed technical devices. The engineering solution ensures well-processed inputs for the problem of coordination of maintenance activities of overhead cranes, which is the object of the study of this research.
KEYWORDS: Monte Carlo Simulation, Markov Chain Monte Carlo (MCMC), Degradation Data Self-Analysis (DDSA), Systems, Applications & Products in Data Processing (SAP), Supervisory Control and Data Acquisition (SCADA), Distributions Algorithm, Integrated Maintenance Platform, Cumulative Distribution Function (CDF)
REFERENCES
Canek Jackson, Rodrigo Pascual. (2021). Joint pricing and maintenance strategies in availability-based product-service systems under different overhaul conditions. Reliability Engineering & System Safety, 216, 107817. - doi:10.1016/j.ress.2021.107817
Deng X., Yanga X., Zhang Y., Lia Y., and Lua Z. (2019). Risk propagation mechanisms and risk management strategies for a sustainable perishable products supply chain. Computers & Industrial Engineering, 135, 1175–1187. - doi:10.1016/j.cie.2019.01.014
Domazet, Z., Luka, F., and Bugarin, M. (2014). Failure of two overhead crane shafts. Engineering Failure Analysis, 44, 125-135. - doi:10.1016/j.engfailanal.2014.05.001
Hanumant P. Jagtap, Anand K. Bewoor, Ravinder Kumar, Mohammad Hossein Ahmadi, Lingen Chen. (2020). Performance analysis and availability optimization to improve maintenance schedule for the turbo-generator subsystem of a thermal power plant using particle swarm optimization. Reliability Engineering & System Safety, 204, 107130. - doi:10.1016/j.ress.2020.107130
Hsu, K., Novara, C., Vincent, T., Milanese, M., Poolla, K. (2006). Parametric and non-parametric curve fitting. Automatica, 42, 1869 – 1873. - doi:10.1016/j.automatica.2006.05.024
Jingyuan Shen, Lirong Cui, Yizhong Ma. (2019). Availability and optimal maintenance policy for systems degrading in dynamic environments. European Journal of Operational Research, 276 (1), 133-143. - doi:10.1016/j.ejor.2018.12.029
Kimiya Zakikhani, Fuzhan Nasiri, Tarek Zayed. (2020). Availability-based reliability-centered maintenance planning for gas transmission pipelines. International Journal of Pressure Vessels and Piping, 183, 104105. - doi:10.1016/j.ijpvp.2020.104105
Lei X. and MacKenzie C.A. (2019). Assessing risk in different types of supply chains with a dynamic fault tree. Computers & Industrial Engineering, 137, 106061. - doi:10.1016/j.cie.2019.106061
Lu, B., Fang, Y., and Sun, N. (2018). Modeling and non-linear coordination control for an underactuated dual overhead crane system. Automatica, 91, 244-255. - doi:10.1016/j.automatica.2018.01.008
Marquez, A., Venturino, P., and Otegui, J. (2014). Common root causes in recent failures of cranes. Engineering Failure Analysis, 39, 55-64. - doi:10.1016/j.engfailanal.2014.01.012
Mori, Y. and Tagawa, Y. (2018). Vibration controller for overhead cranes considering limited horizontal acceleration. Control Engineering Practice, 81, 256-263. - doi:10.1016/j.conengprac.2018.09.009
Naichao Wang, Jiawen Hu, Lin Ma, Boping Xiao, Haitao Liao. (2020). Availability Analysis and Preventive Maintenance Planning for Systems with General Time Distributions. Reliability Engineering & System Safety, 201, 106993. - doi:10.1016/j.ress.2020.106993
Putnik, G.D., Manupati V.K., Pabba S.K., Varela L., Ferreira, F. (2021). Semi-Double-loop machine learning based CPS approach for predictive maintenance in manufacturing system based on machine status indications. CIRP Annals - Manufacturing Technology,70, 365-368. - doi:10.1016/j.cirp.2021.04.046
Qian, D. and Yi, J. (2016). Hierarchical Sliding Mode Control for Underactuated Cranes: Design, Analysis and Simulation. Springer, Berlin. - doi:10.1007/978-3-662-48417-3
Rusinski, E., Iluk, A., Malcher, K., and Pietrusiak, D. (2013). Failure analysis of an overhead traveling crane lifting system operating in a turbo generator hall. Engineering Failure Analysis, 31, 90-100. - doi:10.1016/j.engfailanal.2013.02.008
Sidibé I.B., Khatab A., Diallo C., Adjallah K.H. (2016). Kernel estimator of maintenance optimization model for a stochastically degrading system under different operating environments. Reliability Engineering & System Safety, 147, 109-116. - doi:10.1016/j.ress.2015.11.001
Steurtewagen, B., Van den Poel, D. (2021). Adding interpretability to predictive maintenance by machine learning on sensor data. Computers and Chemical Engineering, 152, 107381. - doi:10.1016/j.compchemeng.2021.107381
Szpytko J. and Salgado Duarte Y. (2020a): Integrated maintenance platform for critical cranes under operation: Database for maintenance purposes. IFAC-PapersOnLine, 53(3), 167-172. - doi:10.1016/j.ifacol.2020.11.027
Szpytko, J. and Salgado Duarte, Y. (2020b). Exploitation Efficiency System of Crane based on Risk Management. In Proceeding of International Conference on Innovative Intelligent Industrial Production and Logistics (IN4PL 2020), 24-31. ISBN: 978-989-758-476-3. - doi:10.5220/0010123200240031
Theissler, A., Pérez-Velázquez J., Kettelgerdes M., Elger G. (2021). Predictive maintenance enabled by machine learning: Use cases and challenges in the automotive industry. Reliability Engineering and System Safety, 215, 107864. - doi:10.1016/j.ress.2021.107864
Wang H., Yan Q., Zhang S. (2021). Integrated scheduling and flexible maintenance in deteriorating multi-state single machine system using a reinforcement learning approach. Advanced Engineering Informatics, 49, 101339. - doi:10.1016/j.aei.2021.101339
Zonta T., Andre da Costa C., Zeiser F.A., de Oliveira Ramos G., Kunst R., da Rosa Righi R. (2022). A predictive maintenance model for optimizing production schedule using deep neural networks. Journal of Manufacturing Systems, 62, 450-462. - doi:10.1016/j.jmsy.2021.12.013
Citation note:
Szpytko J., Salgado Duarte Y.: Degradation Data Self-Analysis Layer for Integrated Maintenance Activities. TransNav, the International Journal on Marine Navigation and Safety of Sea Transportation, Vol. 18, No. 3, doi:10.12716/1001.18.03.14, pp. 601-609, 2024
Authors in other databases:
Janusz Szpytko:
orcid.org/0000-0001-7064-0183
6507258714

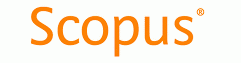