Journal is indexed in following databases:
- SCOPUS
- Web of Science Core Collection - Journal Citation Reports
- EBSCOhost
- Directory of Open Access Journals
- TRID Database - Transportation Research Board
- Index Copernicus Journals Master List
- BazTech
- Google Scholar
2023 Journal Impact Factor - 0.7
2023 CiteScore - 1.4
ISSN 2083-6473
ISSN 2083-6481 (electronic version)
Editor-in-Chief
Associate Editor
Prof. Tomasz Neumann
Published by
TransNav, Faculty of Navigation
Gdynia Maritime University
3, John Paul II Avenue
81-345 Gdynia, POLAND
e-mail transnav@umg.edu.pl
Parameter Identification of Ship Maneuvering Models Using Recursive Least Square Method Based on Support Vector Machines
1 Carl von Ossietzky University of Oldenburg, Lower Saxony, Oldenburg, Germany
2 School of Navigation, Wuhan University of Technology, Hubei, China
3 Institute of Information Technology, Oldenburg, Germany
2 School of Navigation, Wuhan University of Technology, Hubei, China
3 Institute of Information Technology, Oldenburg, Germany
ABSTRACT: Determination of ship maneuvering models is a tough task of ship maneuverability prediction. Among several prime approaches of estimating ship maneuvering models, system identification combined with the full-scale or free- running model test is preferred. In this contribution, real-time system identification programs using recursive identification method, such as the recursive least square method (RLS), are exerted for on-line identification of ship maneuvering models. However, this method seriously depends on the objects of study and initial values of identified parameters. To overcome this, an intelligent technology, i.e., support vector machines (SVM), is firstly used to estimate initial values of the identified parameters with finite samples. As real measured motion data of the Mariner class ship always involve noise from sensors and external disturbances, the zigzag simulation test data include a substantial quantity of Gaussian white noise. Wavelet method and empirical mode decomposition (EMD) are used to filter the data corrupted by noise, respectively. The choice of the sample number for SVM to decide initial values of identified parameters is extensively discussed and analyzed. With de-noised motion data as input-output training samples, parameters of ship maneuvering models are estimated using RLS and SVM-RLS, respectively. The comparison between identification results and true values of parameters demonstrates that both the identified ship maneuvering models from RLS and SVM-RLS have reasonable agreements with simulated motions of the ship, and the increment of the sample for SVM positively affects the identification results. Furthermore, SVM-RLS using data de-noised by EMD shows the highest accuracy and best convergence.
KEYWORDS: Ship Manoeuvering Model, Ship Manoeuvering, Computational Fluid Dynamics (CFD), Extended Kalman Filter (EKF), Recursive Least Square Method, Ship Maneuverability Prediction, Support Vector Machines (SVM), Empirical Mode Decomposition (EMD)
REFERENCES
Skjetne, S., Smogeli, V., & Fossen, T. I. 2004. Modeling, identification, and adaptive maneuvering of Cybership II: A complete design with experiments. IFAC Conference on Control & Application in Marine System (pp.203-208). Ancona, Italy.
Xu, F., Xiao, T., et al. 2014. Identification of Nomoto models with integral sample structure for identification. The 33rd Chinese Control Conference (pp.6721-6725). Nanjing, China.
Åstrom, K., & Kållstrom, C. 1976. Identification of ship steering dynamics. Automatica, 9-22.
Barford, L. A., Fazzio, R. S., & Smith, D. R. 1992. An introduction to wavelets. Hewlett-Packard Labs, Bristol, UK, Tech. Rep. HPL-92-124, 1–29.
Fossen, T. I. 2011. Handbook of Marine Craft Hydrodynamics and Motion Control. Wiley.
Jiang, Z., Yan, W., Jin, X., & Gao, J. 2012. Identification of an Underactuated Unmanned Surface Vehicle. JOURNAL OF NORTHWESTERN POLYTECHNICAL UNIVERSITY, 699-705.
Ljung, L. 2002. Recursive identification algorithms. Circuits, Systems, and Signal Processing, 57-68.
Ljung, L. 2010. Perspectives on system identification. Annual Reviews in Control, 1-12.
Luo, W. L., & Zou, Z. J. 2009. Parametric Identification of Ship Maneuvering Models by Using Support Vector Machines. Journal of Ship Research, 19-30.
Luo, W., & Cai, W. 2014. Modeling of ship maneuvering motion using optimized support vector machines. Intelligent Control and Information Processing (ICICIP), 2014 Fifth International Conference on (pp. 476 - 478). Dalian: IEEE.
Perera, L. P., Oliveira, P., & Guedes Soares, C. 2015. System Identification of Nonlinear Vessel Steering. Journal of Offshore Mechanics and Arctic Engineering, 31302-31307.
Rajesh, G., & Bhattacharyya, S. K. 2008. System identification for nonlinear maneuvering of large tankers using artificial neural network. Applied Ocean Research, 256-263.
Sutulo, S., & Guedes, Soares, C. 2014. An algorithm for offline identification of ship manoeuvring mathematical models from free-running tests. Ocean Engineering, 10-25.
Vapnik, V. 2000. The nature of statistical learning theory. New York: Springer.
Wang, X., Zou, Z. J., & Yin, J. 2013. Modular Parameter Identification for Ship Manoeuvring Prediction Based on Support Vector Machines. The Twenty-third (2013) International Offshore and Polar Engineering (pp. 834-839). Anchorage, Alaska, USA: the International Society of Offshore and Polar Engineers.
Wang, Y.-H., Yeh, C.-H., Young, H.-W. V., Hu, K., & Lo, M.-T. 2014.On the computational complexity of the empirical mode decomposition algorithm. Physical A: Statistical Mechanics and its Applications, 159-167.
XU, F., CHEN, Q., ZOU, Z.-J., & YIN, J.-C. 2014. Modeling of underwater vehicles’ maneuvering motion by using integral sample structure for identification. JOURNAL OF SHIP MECHANICS, 211-220.
Xu, F., Zou, Z. J., Yin, J. C., & Cao, J. 2012. Parametric identification and sensitivity analysis for Autonomous Underwater Vehicles in diving plane. Journal of Hydrodynamics, 744-751.
Zhang, X. G., & Zou, Z. J. 2011. Identification of Abkowitz model for ship manoeuvring motion using ε-support vector regression. Journal of Hydrodynamics, 353-360.
Zhang, X.-G., & Zou, Z.-J. 2013. Estimation of the hydrodynamic coefficients from captive model test results by using support vector machines. Ocean Engineering, 25-31.
Citation note:
Zhu M., Hahn A., Wen Y.Q., Bolles A.: Parameter Identification of Ship Maneuvering Models Using Recursive Least Square Method Based on Support Vector Machines. TransNav, the International Journal on Marine Navigation and Safety of Sea Transportation, Vol. 11, No. 1, doi:10.12716/1001.11.01.01, pp. 23-29, 2017
Authors in other databases:
Man Zhu:
orcid.org/0000-0002-2657-5043

Yuanqiao Wen:
orcid.org/0000-0002-8657-4755
13103950900

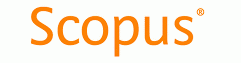
A. Bolles: