Journal is indexed in following databases:
- SCOPUS
- Web of Science Core Collection - Journal Citation Reports
- EBSCOhost
- Directory of Open Access Journals
- TRID Database - Transportation Research Board
- Index Copernicus Journals Master List
- BazTech
- Google Scholar
2023 Journal Impact Factor - 0.7
2023 CiteScore - 1.4
ISSN 2083-6473
ISSN 2083-6481 (electronic version)
Editor-in-Chief
Associate Editor
Prof. Tomasz Neumann
Published by
TransNav, Faculty of Navigation
Gdynia Maritime University
3, John Paul II Avenue
81-345 Gdynia, POLAND
e-mail transnav@umg.edu.pl
Developing Generative Adversarial Nets to Extend Training Sets and Optimize Discrete Actions
1 Kobe University, Kobe, Japan
Times cited (SCOPUS): 1
ABSTRACT: This study proposes the use of generative adversarial networks (GANs) to solve two crucial problems in the unmanned ship navigation: insufficient training data for neural networks and convergence of optimal actions under discrete conditions. To achieve smart collision avoidance of unmanned ships in various sea environments, first, this study proposes a collision avoidance decision model based on a deep reinforcement learning method. Then, it utilizes GANs to generate enough realistic image training sets to train the decision model. According to generative network learning, the conditional probability distribution of ship maneuvers is learnt (action units). Subsequently, the decision system can select a reasonable action to avoid the obstacles due to the discrete responses of the generated model to different actions and achieve the effect of intelligent collision avoidance. The experimental results showed that the generated target ship image set can be used as the training set of decision neural networks. Further, a theoretical reference to optimize the optimal convergence of discrete actions is provided.
KEYWORDS: Maritime Education and Training (MET), MET System in Japan, Unmanned Ship Navigation, Generative Adversarial Network (GAN), Discrete Actions, Lifeboat, Monte Carlo Tree Search (MCTS), Learning Methods
REFERENCES
Capraro, G. T., Farina, A., Griffiths, H. & Wicks, M. C. (2006). Knowledge-based radar signal and data processing: a tutorial review. IEEE Signal Processing Magazine, 23(1), 18-29. - doi:10.1109/MSP.2006.1593334
Finn, A. & Scheding, S. (2010). Developments and challenges for autonomous unmanned vehicles. Intelligent Systems Reference Library, 3, 128-154. - doi:10.1007/978-3-642-10704-7
Goodfellow, I., Pouget-Abadie, J., Mirza, M., Xu, B., Warde-Farley, D., Ozair, S. ... & Bengio, Y. (2014). Generative adversarial nets. In Advances in neural information processing systems (pp. 2672-2680).
Mazurowski, M. A., Habas, P. A., Zurada, J. M., Lo, J. Y., Baker, J. A. & Tourassi, G. D. (2008). Training neural network classifiers for medical decision making: The effects of imbalanced datasets on classification performance. Neural networks, 21(2-3), 427-436. - doi:10.1016/j.neunet.2007.12.031
Mnih, V., Kavukcuoglu, K., Silver, D., Rusu, A. A., Veness, J., Bellemare, M. G. ... & Petersen, S. (2015). Human-level control through deep reinforcement learning. Nature, 518(7540), 529. - doi:10.1038/nature14236
Sarukkai, R. R. (2000). Link prediction and path analysis using Markov chains1. Computer Networks, 33(1-6), 377-386. - doi:10.1016/S1389-1286(00)00044-X
Scheffer, M., Carpenter, S. R., Lenton, T. M., Bascompte, J., Brock, W., Dakos, V., ... & Pascual, M. (2012). Anticipating critical transitions. science, 338(6105), 344-348. - doi:10.1126/science.1225244
Trucco, P., Cagno, E., Ruggeri, F. & Grande, O. (2008). A Bayesian Belief Network modelling of organisational factors in risk analysis: A case study in maritime transportation. Reliability Engineering & System Safety, 93(6), 845-856. - doi:10.1016/j.ress.2007.03.035
Wang, X., Yadav, V. & Balakrishnan, S. N. (2007). Cooperative UAV formation flying with obstacle/collision avoidance. - doi:10.1109/TCST.2007.899191
Citation note:
Zhang R.L., Furusho M.: Developing Generative Adversarial Nets to Extend Training Sets and Optimize Discrete Actions. TransNav, the International Journal on Marine Navigation and Safety of Sea Transportation, Vol. 13, No. 4, doi:10.12716/1001.13.04.22, pp. 875-880, 2019
Authors in other databases:
Ruolan Zhang:
Masao Furusho:
orcid.org/0000-0001-7085-7593
25026052000

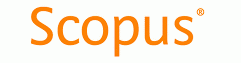